Evaluating Ecosystem-climate Interactions in Eastern Africa
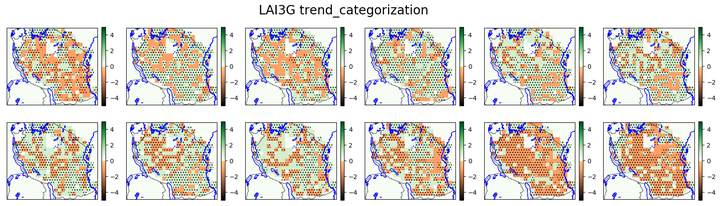
To evaluate complicated ecosystem-climate interactions, I developed novel random forest models tailored for temporal-spatial data, with better prediction accuracy and enhanced interpretability. Classical random forest could not address the spatial heterogeneity and temporal dynamics present in our data. My innovative approach was to fit sub-models on local neighbourhood and further incorporate temporal features, inspired by [1]. We discovered how crucial climate drivers instead of land-use factors are to vegetation trend changes. The ability to model spatial-heterogeneity and temporal trends suggested more effective conservation policies for the protected areas and the great migration.
The stippling plot above shows the regional heterogeity of vegetation trend in Tanzania. The measure of vegetation is Leaf Area Index (LAI) from remote sensing product LAI3G. The trend is decomposed at each pixel level, using data from 1982-2010. Green pixel indicates an increasing LAI trend while brown pixel represents decreasing.
References:
- Stefanos Georganos, Tais Grippa, Assane Niang Gadiaga, Catherine Linard, Moritz Lennert, Sabine Vanhuysse, Nicholus Odhiambo Mboga, Eléonore Wolff, and Stamatis Kalogirou. Geographical random forests: A spatial extension of the random forest algorithm to address spatial heterogeneity in remote sensing and population modelling. Geocarto International, pages 1–12, 2019.